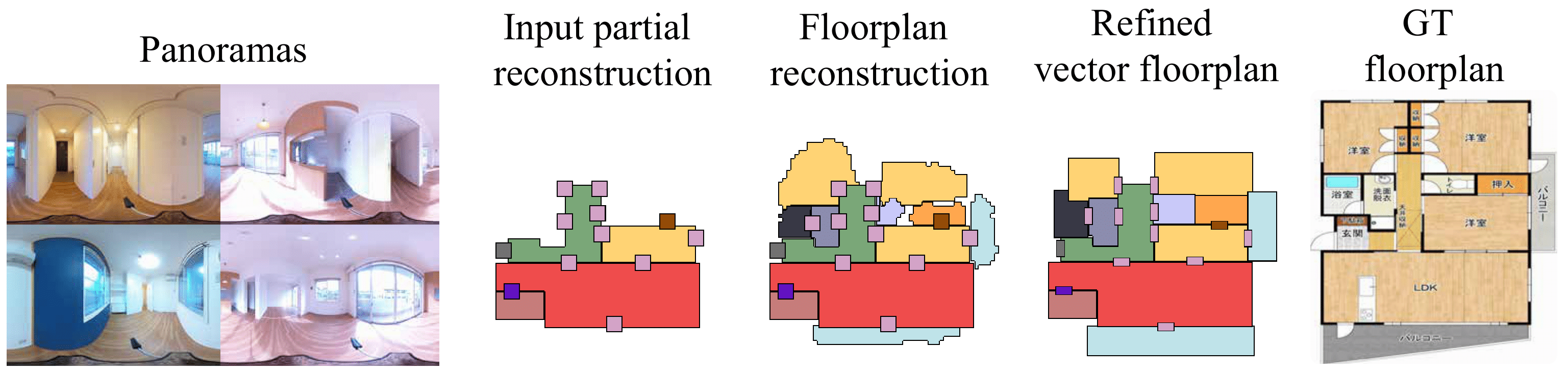
Abstract
This paper presents a novel floorplan restoration task, a new benchmark for the task, and a neural architecture as a solution. Given a partial floorplan reconstruction inferred from panorama images, the task is to restore a complete floorplan including invisible architectural structures. The proposed neural network 1) encodes an input partial floorplan into a set of latent vectors by convolutional neural networks and a Transformer; and 2) recovers an entire floorplan while hallucinating invisible rooms and doors by cascading Transformer decoders. Qualitative and quantitative evaluations demonstrate the effectiveness of our approach over the benchmark of 701 houses, outperforming the state-of-the-art reconstruction techniques. we will publish code and data.

Code and Data
Coming Soon!Citation
@misc{https://doi.org/10.48550/arxiv.2206.00645,
author = {Hosseini, Sepidehsadat and Furukawa, Yasutaka},
title = {Floorplan Restoration by Structure
Hallucinating Transformer Cascades},
month = {May},
year = {2023}}